Co-Authored with Xiq
I (Tasshin) first met Xiq in Portugal in the summer of 2021. We met up in Porto several times with some mutual friends. I was instantly enamored with Xiq’s curiosity, and felt a lot of resonance with him as a friend and peer.
When he made his diagram—a map of high-level interdependence of things that seem relevant in the path from human experience to human flourishing in deep time I was immediately fascinated—and overwhelmed. How did all these ideas fit together?
I invited Xiq to come on my podcast to explain his diagram. He patiently explained it to me from top to bottom, inside out.
Fast forward to January 2024. We met up in the Bay, where I was preparing for my second mettā dance party at the Alembic and Xiq was doing a fellowship at an AI Research group.
We had lunch at a noodle bar and went on a long hike. A lot of our conversation that day was about Active Inference and the Free Energy Principle (FEP). Xiq had mentioned it to me before, but I hadn’t really understood it. A friend had sent me some articles about it but I hadn’t read them—they didn’t really seem like they met me where I was, or on terms that would help me digest the ideas. Xiq, on the other hand, was well positioned to clearly explain the ideas to me in a manner and context that I could grasp.
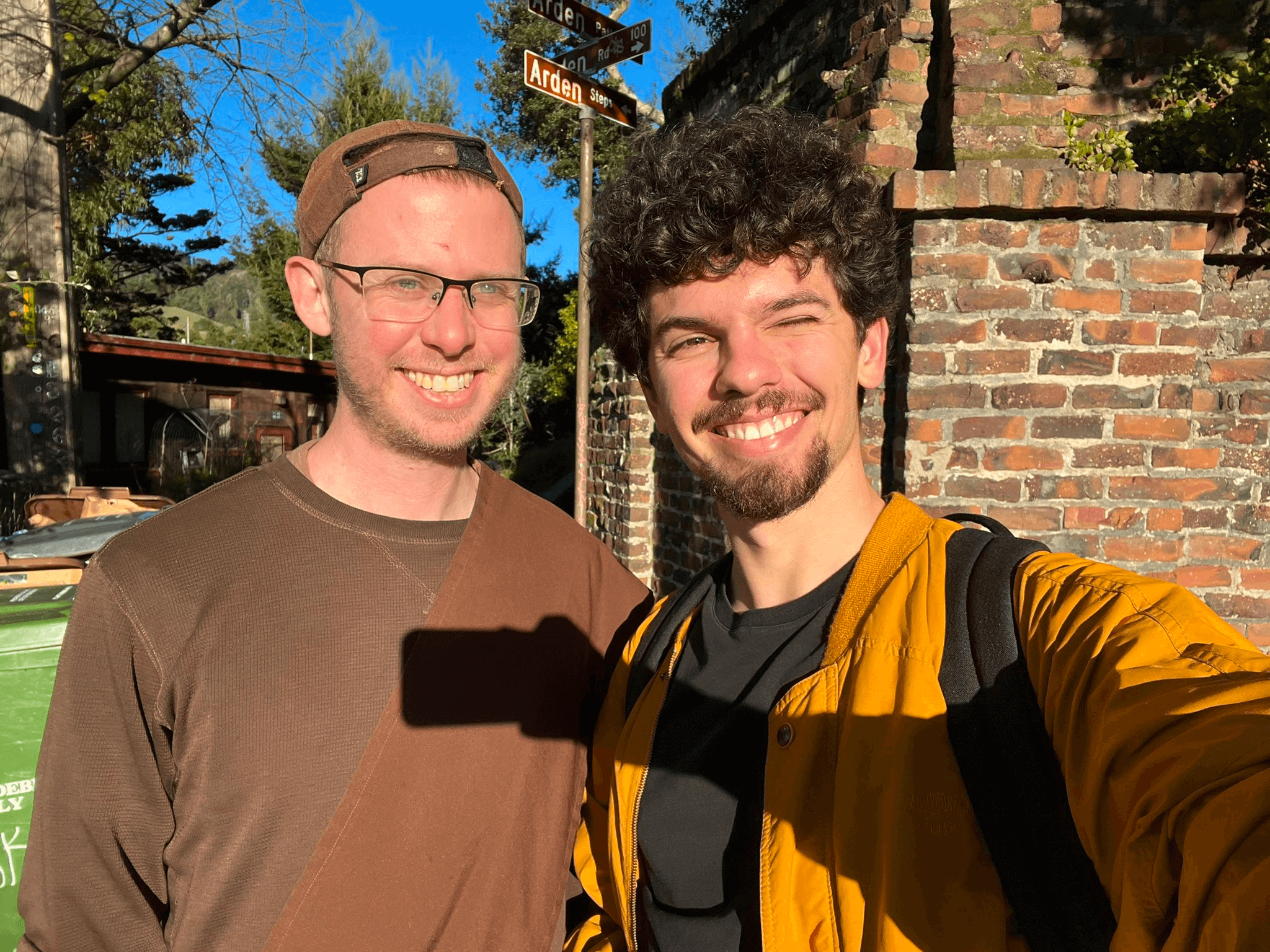
I peppered Xiq with hundreds of questions. I asked him to explain specific ideas in simple terms, and asked how they connected to each other. When I didn’t understand something, I pushed back and asked him to try again. It was exhilarating.
Once I got the basic gist of the Free Energy Principle, I saw that it had tremendous explanatory power about… nearly everything. I asked Xiq again and again how it related to different ideas I was interested in: God, awakening, mettā, social behavior, psychology, ethics… I delighted in his answers, and even began to share a few of my own.
Xiq’s Story
I have always had diverse interests: Pokémon, visual art, computers, music, physics, AI, history, linguistics, governance, meditation, EU policy, religion, etc. These often felt disconnected, and I’ve wanted to fit them together, to see how they relate.
That’s why I’ve always been fascinated by big ideas and theories of everything. I wanted to find ideas that explained how things were connected across contexts and at different scales: cells, organs, sub-personalities, individual people, families and teams, congregations and companies, cities, countries and networks etc.
It’s not that they all map neatly, but there are principles that apply across scales and domains. This is the case in network science—where properties hold in networks of all kinds: social, telecommunications, ecological, etc—or the notion of computation, which at its most general is about change and problem solving—or information theory, which is about patterns, probability, and uncertainty.
Over time, I gathered different frames and tried to apply them to connect different ideas I was interested in. These frames included computation, network science, and information as stated above, as well as emptiness, dependent arising, game theory, and machine learning.
These analogies between domains seemed to suggest an underlying language to talk about entities and their interactions across scales, but for a very long time I couldn’t quite articulate it. Over time, I kept spotting something called “the free energy principle,” which had a distinct information theory flavor. I saw it applied to very diverse settings and thought “this could be it!”
The FEP is famously hard to get into, but after a few waves of “run into it; read a few explainers; forget about it; repeat”—I think it stuck well enough that I could talk about it. I’ve since felt like I needed to up my game to write this post so I ended up going deep on Parr’s Active Inference book, as well as other people’s ways of explaining it.
Overview
This post will explain the basic ideas of Active Inference, Predictive Processing, and the Free Energy principle. It won’t be a thorough, academically rigorous explanation, but rather it is intended to be an approachable, understandable, accessible introduction to these ideas.
Furthermore, we’ll share basic, hand-wavey suggestions about how these frames apply to ideas we are both interested in—again, not in a rigorous way, but in an evocative way that might be fleshed out further at a later date, or will help you to see how these ideas apply to others that you care about.
Introducing Active Inference and the Free Energy Principle
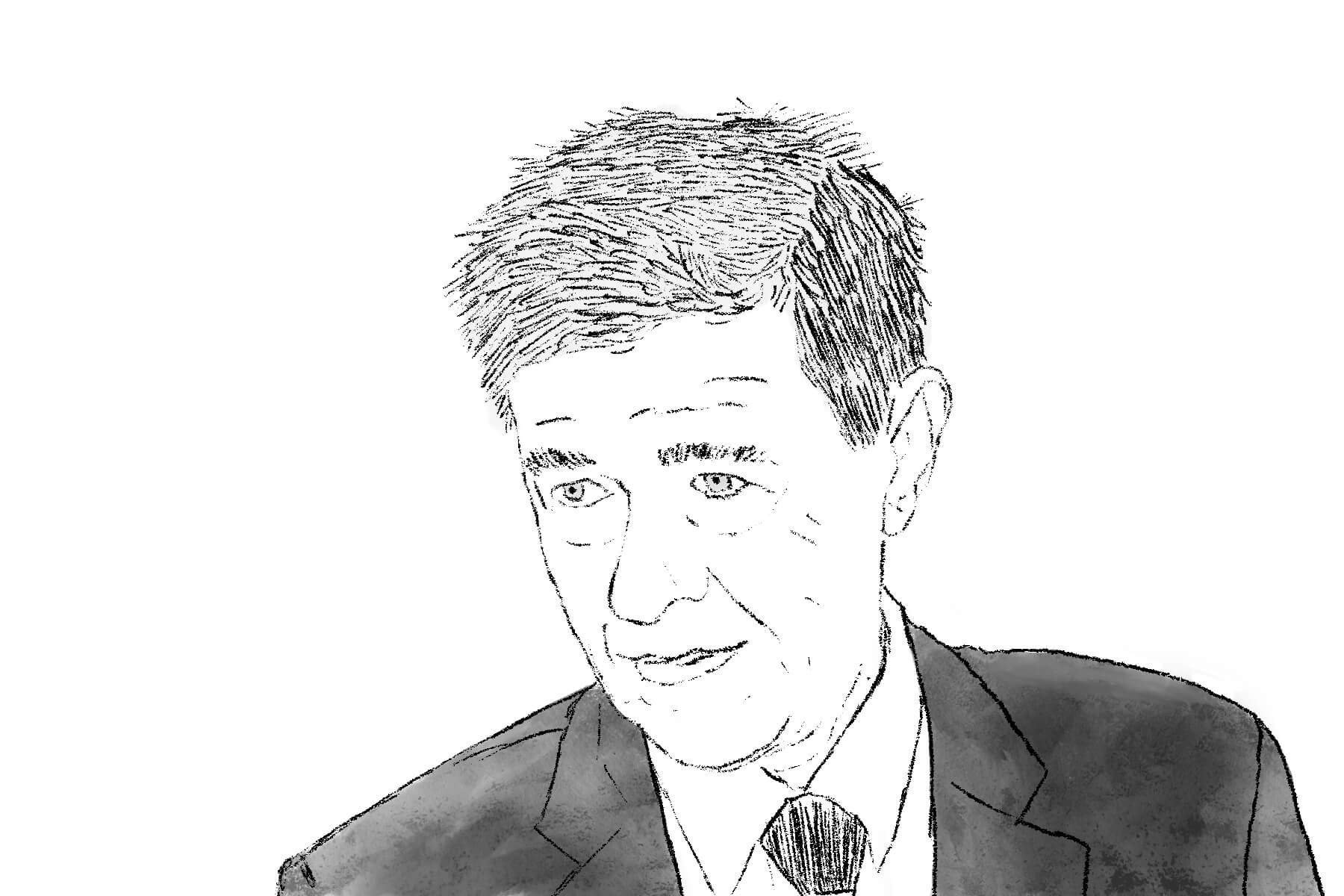
Karl Friston is a British neuroscientist and theoretician at University College London. He is an authority on brain imaging and theoretical neuroscience, and a key architect of the Free Energy Principle and Active Inference (ActInf). Friston was curious about the principles underlying brain function and neuronal interactions, and that led to the development of his theories.
To attempt a brief explanation of active inference:
The world is full of living organisms that act upon the world to change it, and receive sensory observations from it.
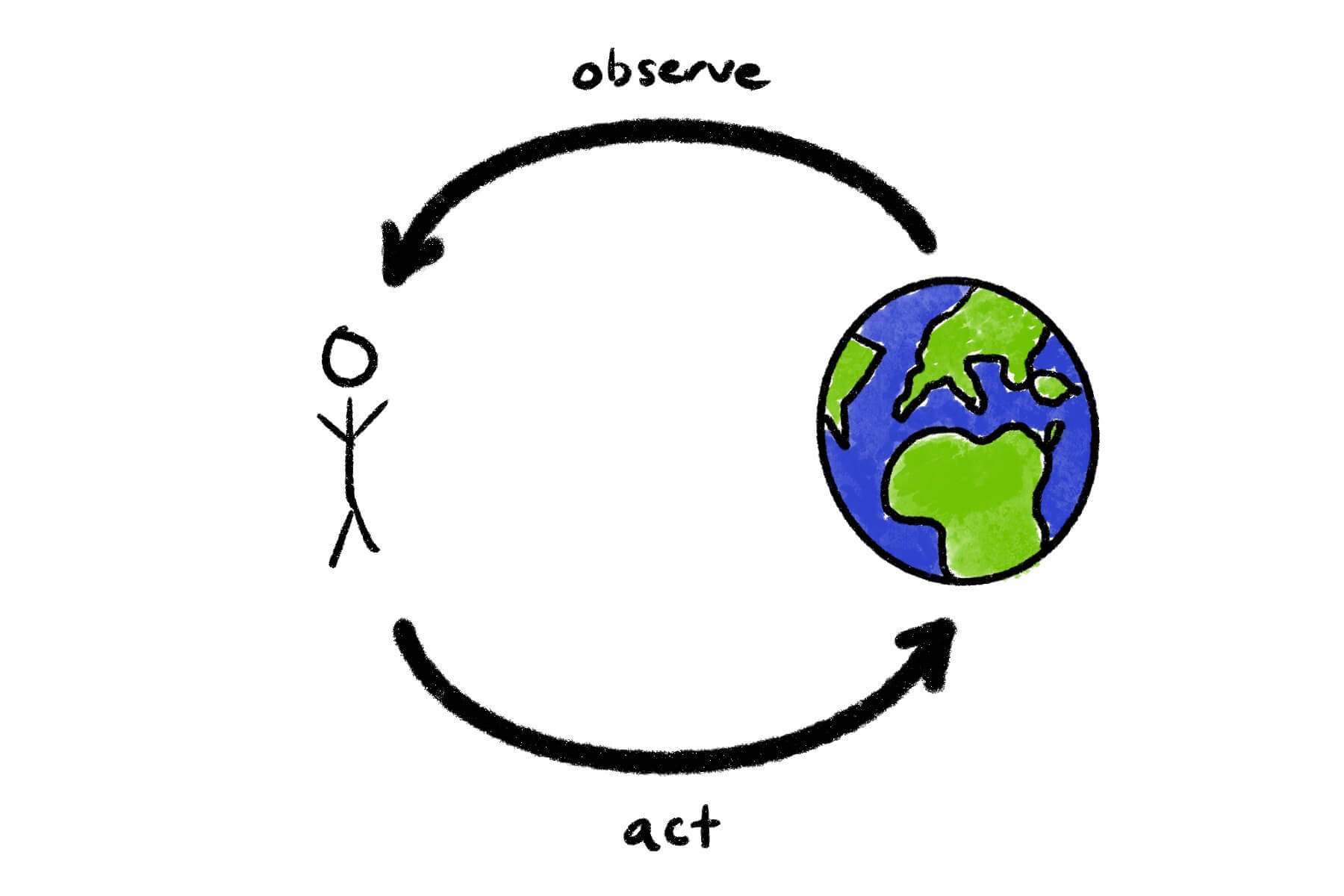
They stay alive by adaptively controlling their exchanges with the world. These strategies can be as simple as following nutrient gradients in bacteria, or as complex as humans planning to achieve future goals. These strategies also vary for different timescales—ranging from evolutionary adaptation, to cultural and developmental learning, to real-time action and perception.
Given this diversity, it may sound surprising that all these kinds of behavior and cognition could be explained from first principles. Active Inference is a framework that explains perception, action, learning, and planning (and other stuff) in adaptive organisms as following a unique imperative: the fundamental drive of self-organizing systems is to minimize surprise from their sensory observations.
Self-organizing systems include cells, brains, and economies. We can describe these as things that don’t dissipate into the background – or things that maintain homeostasis. A drop of ink in a water cup dissipates, whereas a cell, a tree, or an anthill do not. Things usually have boundaries like cell walls, skin, national borders, which can be formalized as Markov blankets.
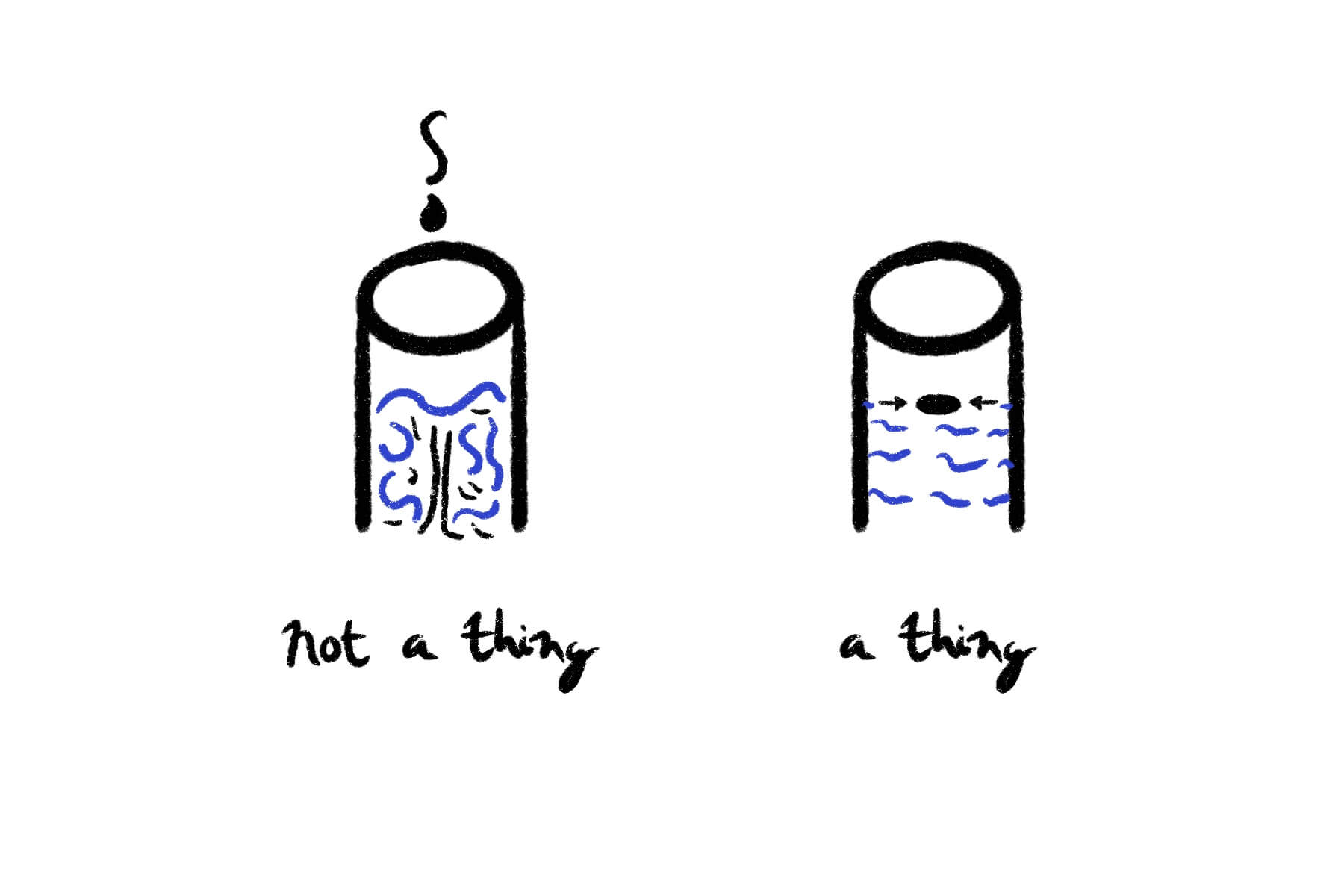
A drop of ink not dissipating would be weird. It would make it a “thing”.
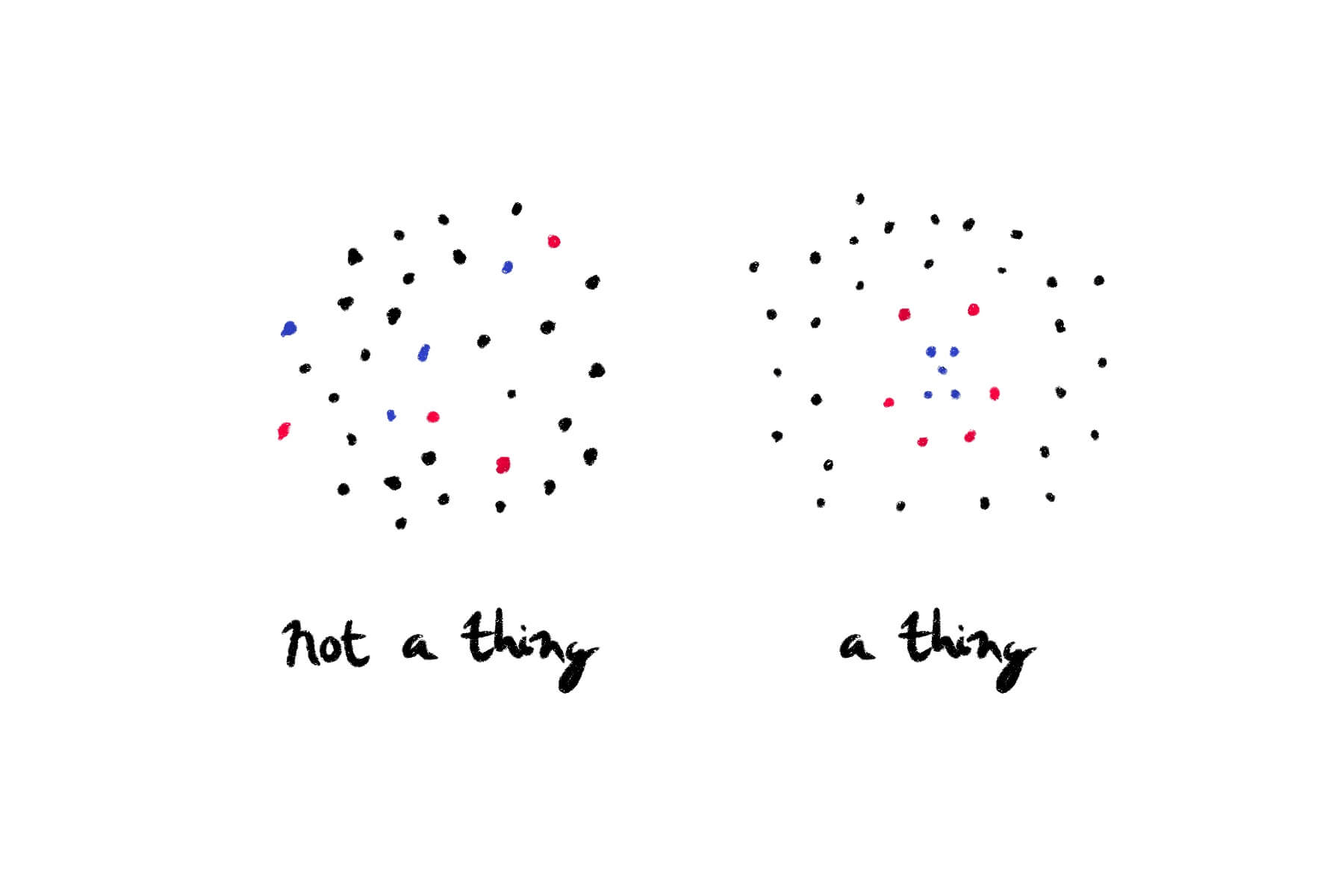
Imagine a primordial soup of particles. If they’re mixed, you can’t really tell things apart. But if you see an arrangement of them persist through time as the environment around it changes, you would say that’s something.
By surprise, we don’t mean the conventional sense of the word. We mean the divergence between one’s expectations (or predictions), and one’s sensory observations. This quantity is also known as prediction error.
Surprise is destabilizing. And in this framing, destabilization is surprise. Destabilizing events knock you out of homeostasis (kill you). So you either want to get good at anticipating danger in complex surroundings, or to change your environment to one that supports your homeostasis.
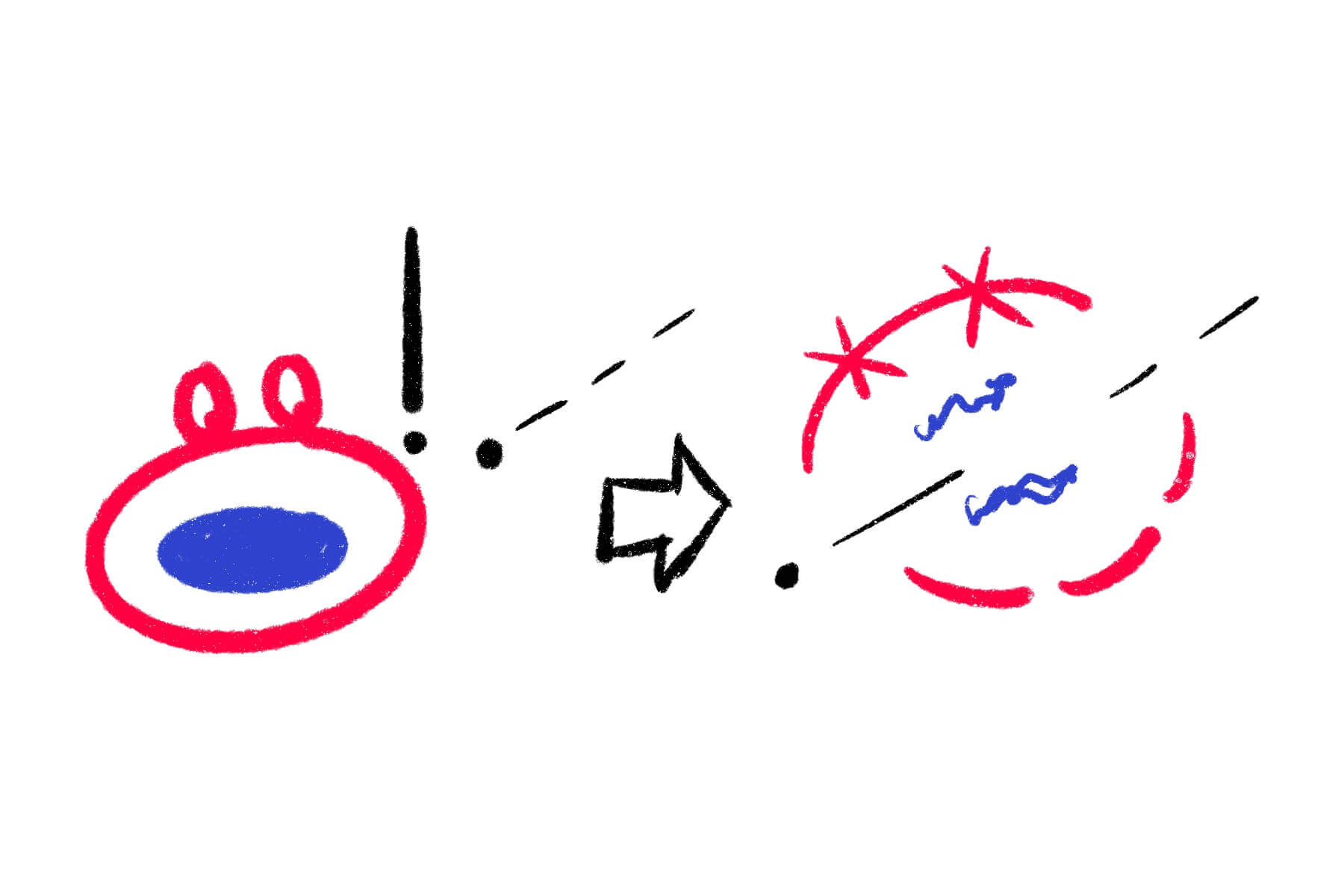
A cell is surprised by an incoming projectile and destabilized (dead).
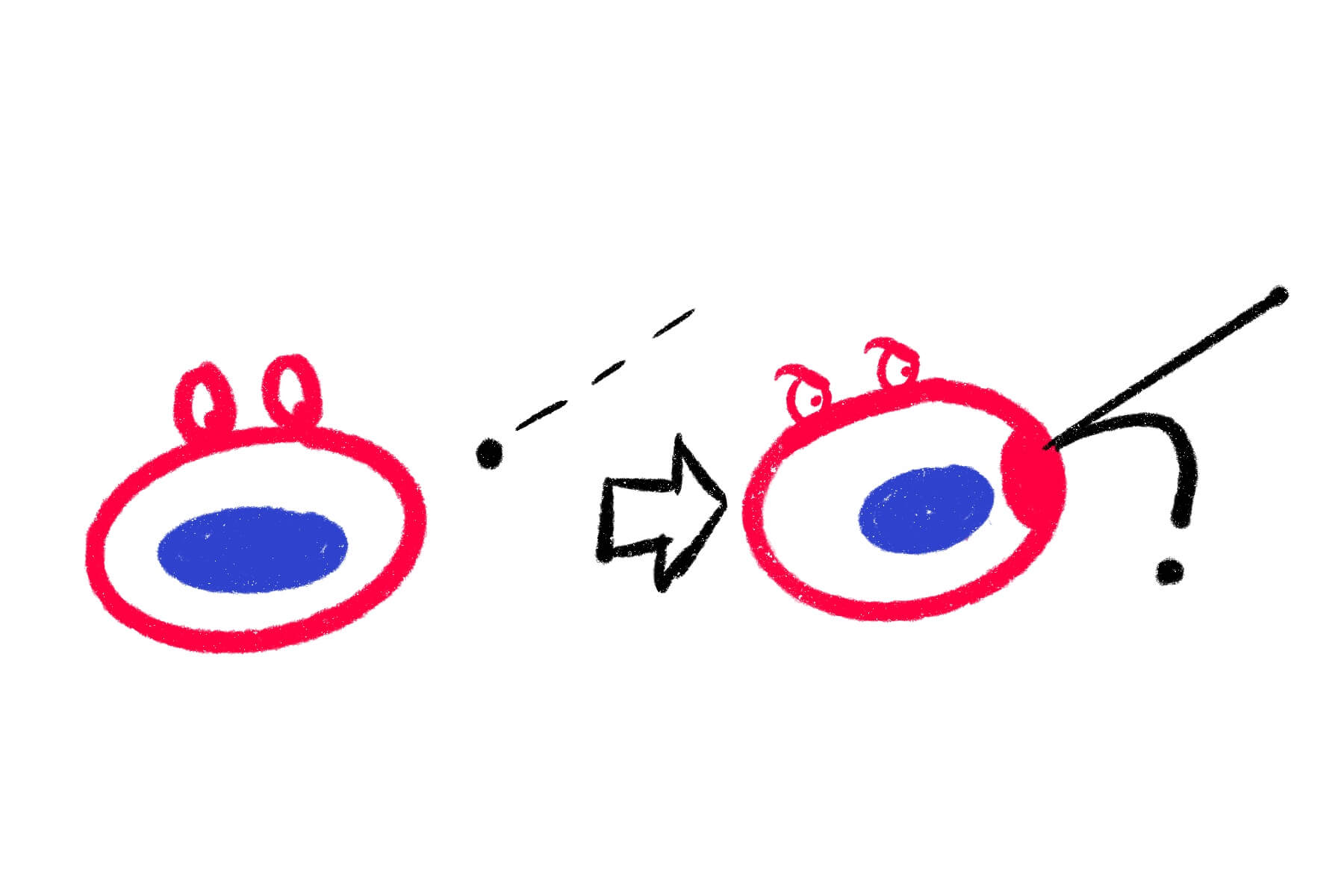
A cell anticipates an incoming projectile and hardens its boundary (acts) to defend itself and remain in homeostasis (alive).
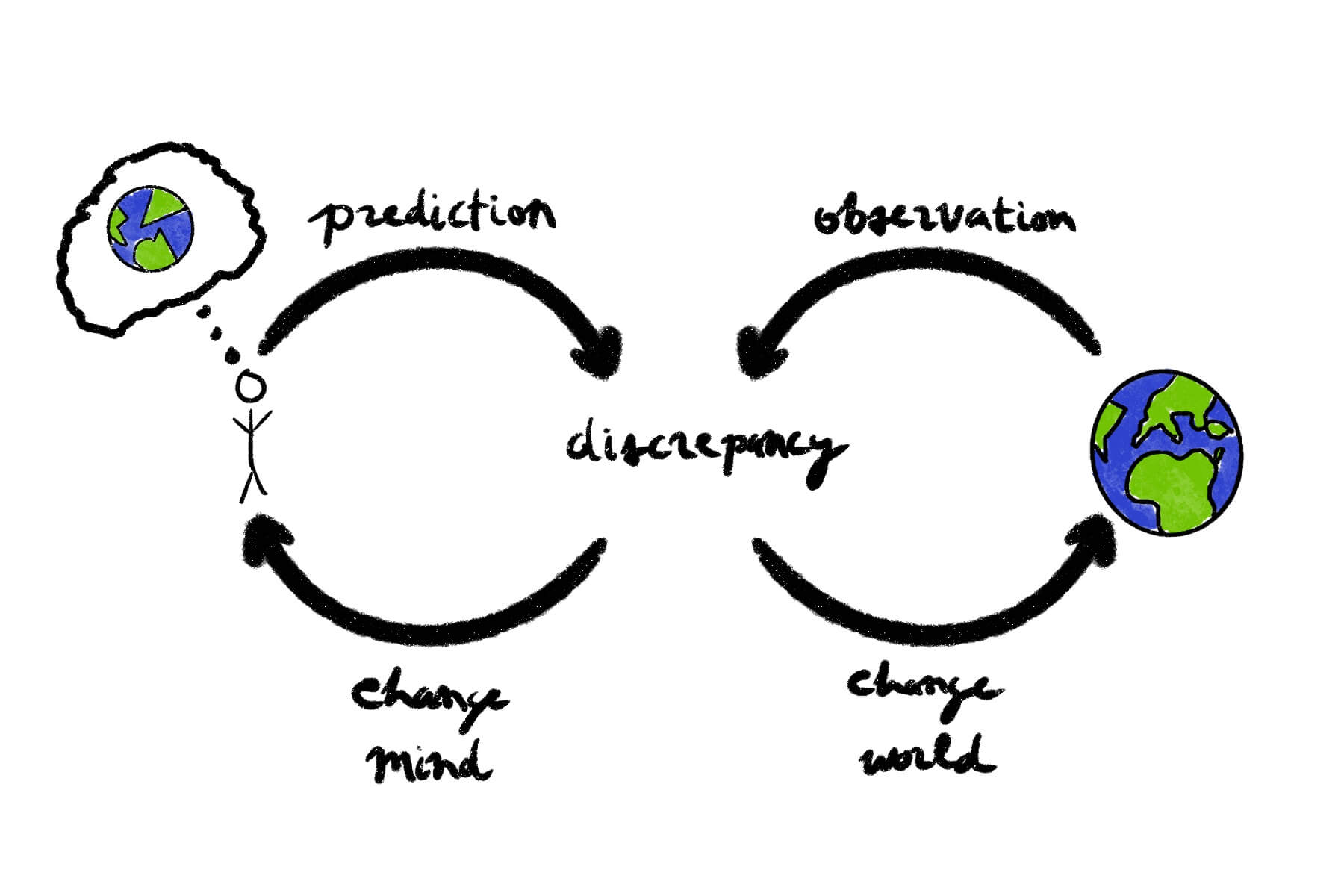
When you’re surprised, you can either change your mind (update your beliefs) or change the world (act).
“Isn’t surprise good? Isn’t it valuable to actively seek surprising information or novelty?,” you might say. We minimize surprise over time, so we’re trading off exploration (information gain) and exploitation (utility, having the world match our expectations). Anticipating danger requires mapping the unknown to better predict the world in the future, so you’ll want to gather information about it first. Same for changing your environment: you need to learn about how the world behaves if you want to be able to predict the consequences of your actions.
Or maybe you’d say, “If we’re minimizing surprise, why don’t we lock ourselves in a dark room?” A dark room just isn’t an environment that supports your homeostasis very much. It’s not somewhere you’re adapted to. So you would feel compelled to turn the light on, to gather information and change your environment for the better.
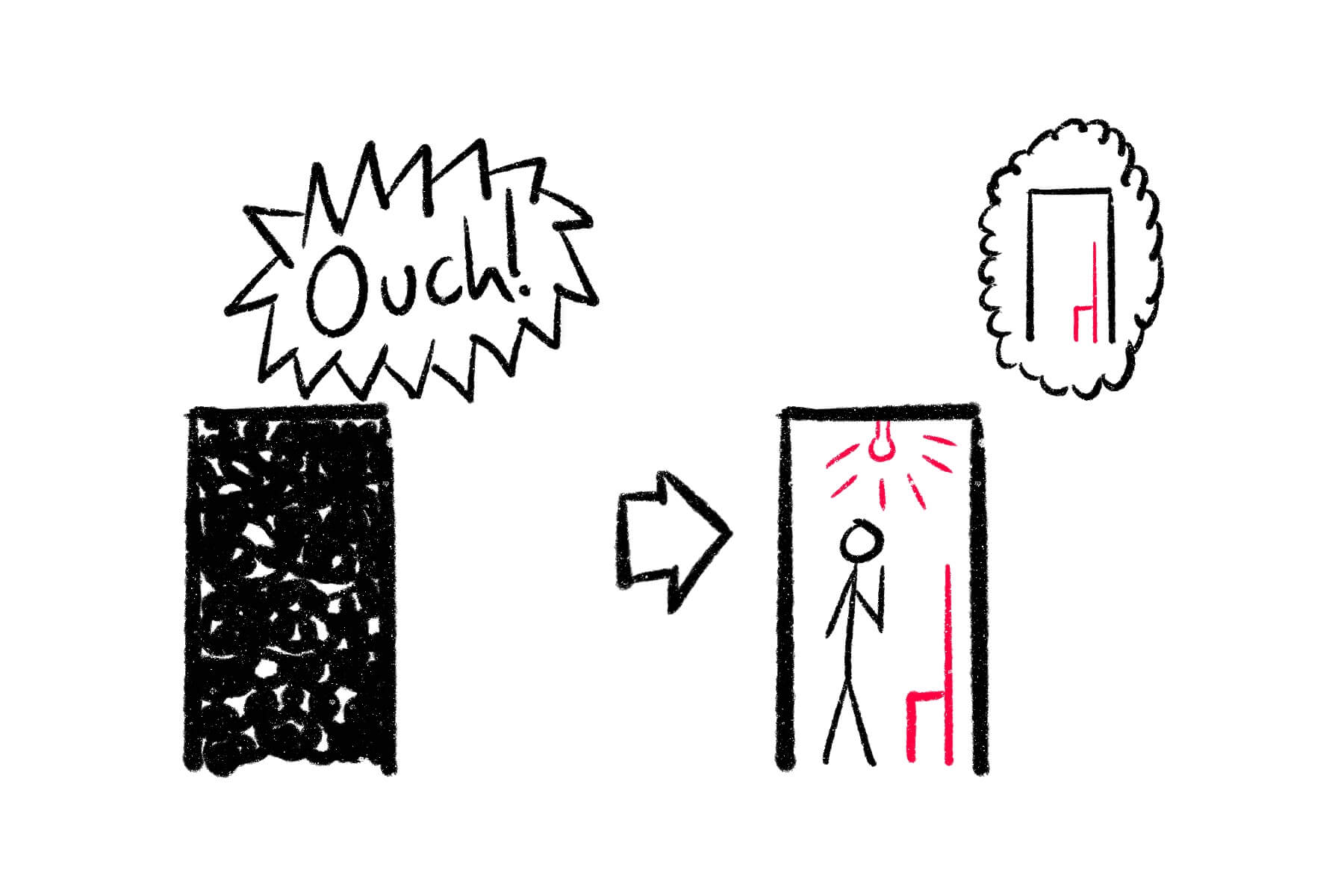
If the light is off, you have no information about your environment and are consistently surprised by bumping into things. When you act to turn the light on, you get new information and update your model of the world, so you won’t be surprised by where things are anymore.
By an environment that supports homeostasis, I mean one that satisfies the conditions needed for survival (i.e. to not dissipate) like the right temperature range, available food and drink, and no physical danger. We can stretch this concept of homeostasis to include our preferences and tastes, so in a minimally surprising environment I’d be surrounded by loved ones, and by art and stimuli perfectly suited to my current mood.
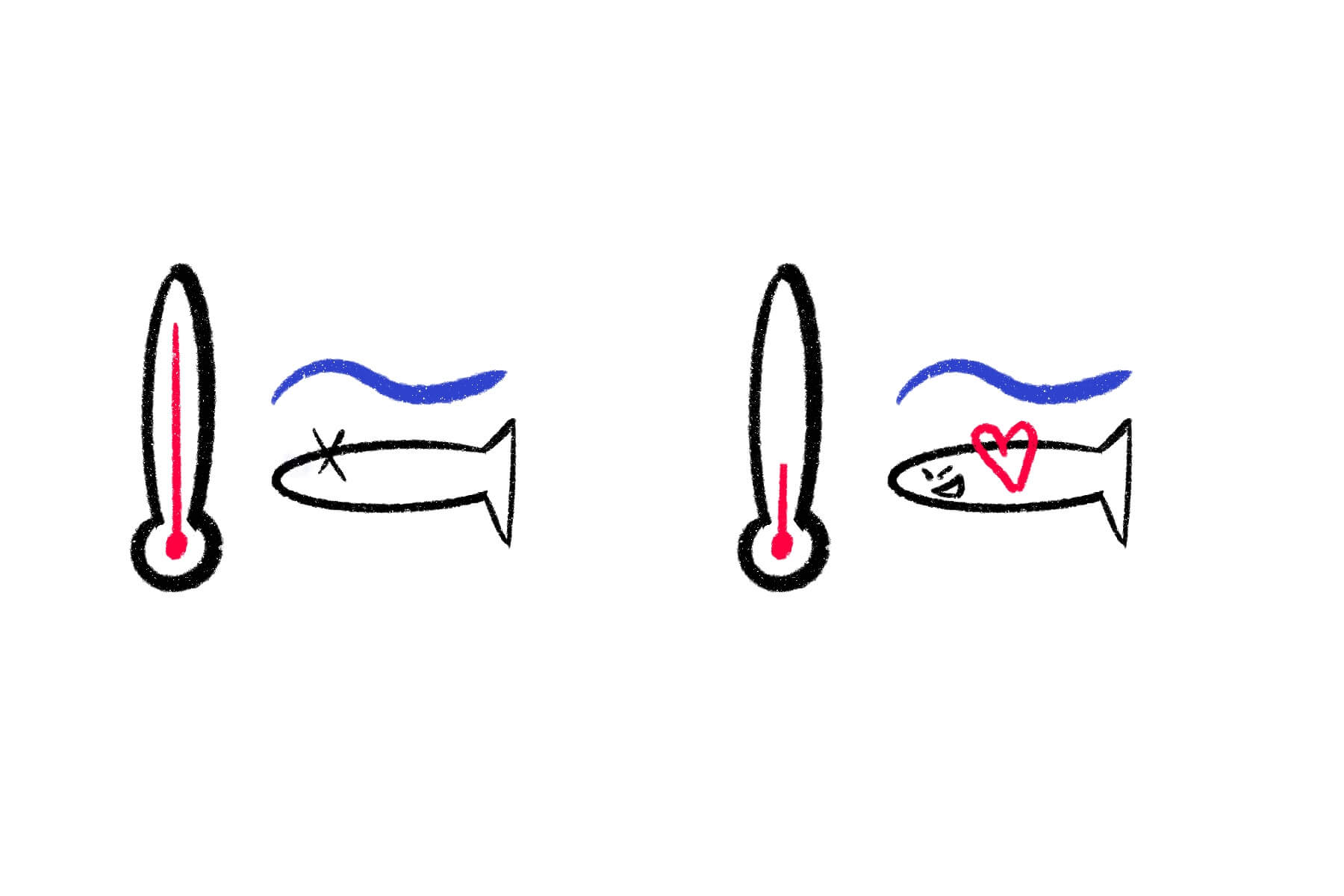
The fish needs a certain water temperature to support its homeostasis (ie. not dying, remaining a thing). That temperature is part of the fish’s existential preferences.
Some other terms like “Active Inference,” “Bayesian brain,” and “predictive processing” are also frequently associated with the FEP. I (Xiq) think this diagram from Michael Edward Johnson’s Seed Ontologies does a good job of distinguishing between them:
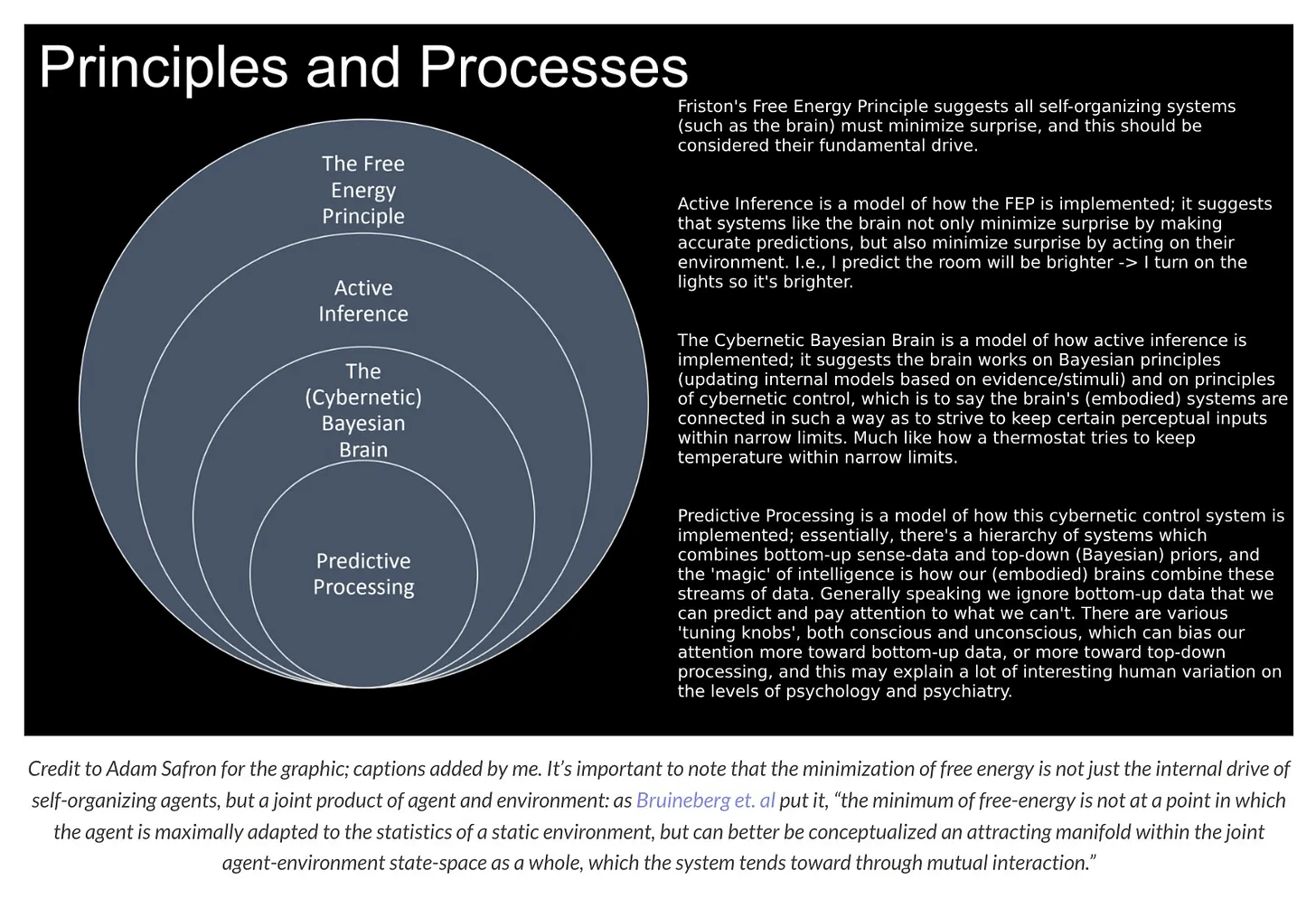
Active inference assumes these “ideal conditions for homeostasis” are encoded in organisms as implicit beliefs about themselves, and calls them “preferences.” (In Bayesian terms, preferences are a subset of an organism’s generative model’s priors.)
Preferences account for a lot, because we resort to them to explain all survival-related and goal-directed behavior, as well as our open-ended search for comfort and beauty and so on. To get more insight, you could ask where preferences come from, how they’re put in place by evolution and culture and learning, and people do ask those questions.
You could question the utility of active inference given that so much bottoms out in preferences and those are still being figured out. My opinion is that it’s better to have a simple coherent framework where one of the components is pretty complex, than it is to have a system that is very complex or disorganized at the top level.
Philosophical Status of the FEP
This “summary for a wide audience” helped me by clarifying that the FEP is not a theory: it’s a principle based on which we can formulate theories that partition a system into “things” that are separate from but coupled to others by beliefs. Just like the principle of least action gives rise to classical mechanics in Physics and lets us predict motion in systems of rigid particles, the FEP lets us predict behavior in systems of things that model each other.
Glossary
Term | Definition |
Free Energy Principle (FEP) | A theoretical framework that describes how living systems maintain order and resist entropy by minimizing free energy, which is related to surprise or prediction error. |
Active Inference | A process by which organisms minimize free energy by continuously updating their models of the world through perception and action. |
Bayesian Brain | The hypothesis that the brain functions as a Bayesian inference machine, constantly updating its beliefs based on sensory input. |
Predictive Processing | A theory suggesting that the brain continuously generates and updates a model of the environment to predict sensory input and minimize prediction errors. |
Homeostasis | The process by which a system maintains internal stability despite external changes. |
Markov Blanket | A concept from statistics defining the boundary that separates the internal states of a system from external states, allowing interaction without dissolution. |
Surprise (in FEP context) | An information-theoretic quantity representing the difference between expected and actual sensory input; minimizing surprise helps maintain stability. |
Bayesian Inference | A method of statistical inference in which Bayes’ theorem is used to update the probability of a hypothesis as more evidence or information becomes available. |
Variational Free Energy | An approximation of free energy used in Bayesian inference. Without an approximation, Bayesian inference is intractable (takes too long to compute). It balances model complexity with accuracy. |
Expected Free Energy | The predicted variational free energy over time, guiding decisions that balance exploration and exploitation to minimize future surprise. |
Generative Model | A model used to generate predictions about sensory inputs based on internal states and beliefs. |
Hamiltonian Principle of Least Action | A principle in physics stating that the path taken by a system between two states is the one for which the action is minimized. |
Entropy | A measure of disorder or randomness in a system, often related to the second law of thermodynamics. |
Epistemic Value | The value derived from reducing uncertainty or gaining knowledge about the world. |
Pragmatic Value | The value derived from achieving goals or fulfilling practical needs. |
Projection
One of the things learning about Active Inference helped me (Tasshin) to understand was psychological projection. Psychological projection is when people judge other people for their undesirable thoughts, feelings, and behaviors which are really their own qualities. I’d been curious about projection since 2019, when I was reflecting a lot on psychology and human nature, and was working on my ability to read people.
I saw other people projecting things onto others, and, when I was honest with myself, I could see myself projecting, too. Projection seemed like a basic fact of human experience to me—I completely accepted it as a fact of human psychology—but I couldn’t understand how it worked, exactly, or precisely why it happened.
I did some of my own research, and went in search of explanations. I checked with my friends in person who seemed knowledgeable, and asked around on the internet. The explanations I encountered weren’t very satisfactory. Most of them seemed circular to me: explaining projection by way of projection’s existence.
Five years later, I was still curious about this. I hadn’t found any explanations that really satisfied me. I asked again on Twitter. This time I got some different and slightly more elaborate answers, but they still weren’t very satisfying.
Xiq and I had just recently had our extensive conversation about Active Inference. It suddenly occurred to me that Active Inference might hold the clue I was looking for.
From the perspective of The Free Energy Principle and Active Inference, people are constantly modeling the world and each other. They base their models on experiences they’ve had, and information they’ve already encountered. In the absence of better information, people will model others based on themselves: “Hey, all people are people-shaped, and I’m people-shaped, so they’re shaped like me, too, right?”
This is an efficient way to establish models of other people, because everyone has large amounts of information about themselves. We all have direct access to all our own memories, experiences, preferences, judgements, etc. But the tendency to model others based on ourselves sets us up to be surprised, because in fact, other people are not exactly like us. The world is broader than our experience.
From the perspective of the FEP, projection can be seen as incorrect predictions about others, because we overfit on models of ourselves. It can be counteracted by cultivating theory of mind, which helps to diminish surprise.
This was an instance of something genuinely puzzling to me—where the existing explanations that I’d encountered did not satisfy me, but the Active Inference frame did feel satisfactory. It also gave more color and force to existing explanations that I’d encountered, e.g. “limited cognitive resources.”
The point here isn’t that I’d learned everything about projection, or solidly proved something logically to the whole world. It was that I had finally found an explanation that satisfied my own curiosity and mind. As Xiq points out, other frameworks that involve modeling one’s environment could probably be similarly descriptive. But for me, Active Inference and the FEP provided a sufficiently satisfying explanation that closed the books on a question I’d been curious about for literally years.
Viewing Concepts Through A Lens of Active Inference & Free Energy
epistemic status: we had too much espresso and are gesticulating wildly over our noodles
Once Xiq had explained the basics of Active Inference and the Free Energy Principle, I (Tasshin) asked him how he thought these ideas might apply to a number of different ideas. In this section, we (mostly Xiq) have come up with a number of these explanations for specific ideas. We are mostly guessing here, doing “conceptual jazz,” and playing “What If?”.
These definitions may be somewhat unhelpful in illustrating the FEP since they end up folding in a lot of my (Xiq’s) idiosyncratic philosophy. I did try to make these terms mostly match the ways they’re used historically, so I hope it won’t be too bad.
Term | Synthesis |
Curiosity | Curiosity signals potential information gain, driving us to complete our mental models and reduce future surprise. For example: turning on a light in a dark room, so that we can better understand the space and what’s in there. |
Fun | Fun is closely related to the flow state, which John Vervaeke defines as being in the zone of proximal competence, under the following conditions: clear information, tightly coupled feedback, and error mattering. I visualize fun as surfing the optimal balance of novelty and familiarity, of competence and challenge, of safety and danger, explore and exploit. |
Cooperation | Cooperation and coordination lower surprise by aligning expectations and actions among individuals. For example, in the case of multicellular life, only the outside cells need to contend with the outside world – all the interior cells have a much lower-surprise environment consisting of other cells like them. |
Integrity | Integrity is a prosocial policy that creates a stable, reliable social environment by minimizing unexpected surprises. When people consistently do what they say they will do, it fosters predictability and trust in social interactions. |
Etiquette | Etiquette involves behaving in ways that avoid surprising or discomforting others unnecessarily. For example, moving smoothly or tailoring speech to others’ comprehension levels both reduce uncertainty and potential discomfort in social situations. |
Community | Communities are like a multicellular organism made up of humans. A Markov blanket forms around the group. Different members take different roles handling various aspects like food sourcing, diplomacy, and protection, so that not everyone needs to interact with every part of the environment. Communities minimize surprise for individuals because they reduce surface area with an unpredictable environment. |
Magick | Magick can be seen as architecting outcomes by inducing certain expectations in oneself and/or others, leading to actions that conform to those expectations. It’s most effective when the induced, load-bearing beliefs are difficult to falsify. Then you can generate a narratively compelling hypothesis that isn’t immediately updated from contact with the world. |
Manifesting | Manifesting involves cultivating a clear sense of readiness and worthiness for desired outcomes, which influences one’s actions and interactions. This creates a latent information imprint that can subtly guide opportunities and resources towards you through the distributed intelligence of the social network. |
Service | Service recognizes one’s dependence on the larger social organism, which justifies seemingly altruistic actions as self-beneficial. Using one’s knowledge to help others minimize surprise in their environments, contributing to the overall well-being of the community, and ultimately benefits you, because you’re contributing to and part of the same thing. |
Community Organizing | Community organizing is a form of service. It’s like doing social permaculture: applying tricks here and there that strive to establish wholesome norms, and nudge the system in positive directions. It involves bringing people together, facilitating information exchange, and creating environments conducive to forming relationships and fostering community-building behaviors. |
God / Superorganism | One way to understand God is as a principle (love) implemented through individual actions, resulting in desirable emergent conditions. God as an adaptive coordination mechanism upheld by faith—faith that if everyone cooperates, things will be great and we’ll live in the kingdom of God. The emergent superorganism self-organizes to provide for the virtuous and punish defectors. |
Serendipity / Grace | Grace is reducing the degree of effortful involvement while maintaining or improving results. For example, changing your environment rather than constantly adjusting behavior; setting intentions and allowing things to unfold naturally; cultivating rather than hunting all the time—moving towards a more receptive (yin) approach to life. |
Awakening / Spiritual Enlightenment | One way we can guess at conceptualizing awakening is as defaulting to the flow state. You learn your own body-mind dynamics so well that you spend way less energy on operating yourself and are able to invest much more in interacting with the world. Ideally, you’re always at the optimal point between challenge and ability, hitting playful engagement without overexertion, resulting in maximally efficient resource management and a discrete shift downwards in free energy baseline. |
Buddhist Right View and Wrong View | Is Wrong View just “being wrong about how things work, especially w/r/t the origin and propagation of dukkha up the scales of complexity?” is Right View just “having good models of how things work”? |
Insight Meditation | Insight meditation is learning about one’s own mind to create a more accurate predictive model of oneself, and spend less energy dealing with the errors introduced by your own machinery. |
Concentration Meditation | Concentration meditation trains sensory clarity, allowing perception of finer and briefer phenomena. This enhanced perceptual ability enables detection of subtler divergences between prediction and perception, leading to further optimization of mental models. |
Mindfulness | Mindfulness cultivates subtle and broad awareness, letting you pick up on information that helps you refine your models. Stress patterns and blind spots are like frozen priors that don’t update, which can be unfrozen by irrigating them with attention. |
Memory Reconsolidation and Self-Therapy | Self-therapy techniques often focus on accessing and updating “frozen priors”—deeply held beliefs resistant to change. These can be hard to reach, because part of what they do is leading you to wrongly predict that you’ll be in danger if you touch those priors. Insight and mindfulness can reveal where to intervene and how to do it gracefully. |
Mettā (Loving-kindness) | Mettā embodies the principle of oneness with the superorganism, recognizing all conscious beings as fundamentally connected, on the same team. We wish for their happiness, freedom from suffering, low free-energy (surprise, discomfort). Access to mettā enables us to ride the evolutionary advantage of cooperation and coherence. We have access to a feeling, a resonant mode of the nervous system, that directs gregarious action. |
Karuṇā (Compassion) | Karuṇā is compassionate perception and action, aimed at minimizing suffering or free-energy in others. It’s a natural outgrowth of mettā. |
Muditā (Sympathetic joy) | Muditā involves identifying with the superorganism to the extent that others’ well-being brings us joy. |
Upekkhā (Equanimity) | Upekkhā involves minimizing the “second arrow” of suffering by quickly stabilizing our system after a stimulus, rather than amplifying it and letting it perturb subsequent processing. By reducing the turbulence of our own state and the noise in our perception, it makes our internal and external experiences more predictable and manageable. |
Conclusion
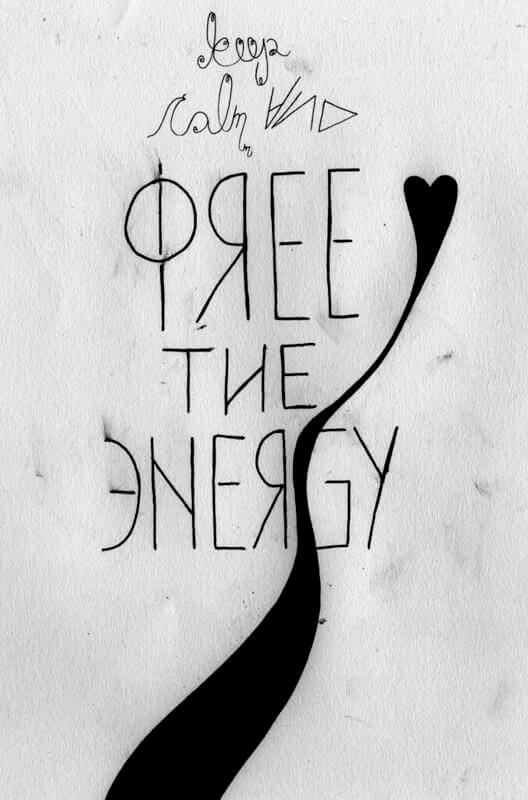
Keep Calm and Free the Energy! by Daniel Friedman (CC BY-NC 2.0)
Hopefully we were able to give you a sense of the free energy principle’s utility as a lens. We can use it to make quick and dirty sense of things, but we can also pursue rigor with more effort. If we so wished we could use the same thinking style, the same conceptual “shapes”, and ground our insights with math in a physics of information coupling between interacting things.
May our shared desire for knowledge about the universe and harmony in life, driven by the natural principle of minimizing free energy, manifest as deep, unconditional love for and cooperation between all beings. ❤️
Further Resources
As we’ve shared, our intention for this post was to introduce them in an approachable way at the expense of some rigour, and to give a sense of their applications and thus why we are so passionate about them. If you’d like to dive deeper into Active Inference and the Free Energy Principle, here are some resources you could go into to explore more deeply.
Wired’s Shaun Raviv did a really lovely profile of Karl Friston and his ideas, The Genius Neuroscientist Who Might Hold the Key to True AI. Here are some his formal papers where he shares his theories:
- The Free Energy Principle made simpler but not too simple by Friston et al.
- The free-energy principle: a unified brain theory? by Karl Friston
- A free energy principle for the brain by Karl Friston, James Kilner, and Lee Harrison
The Active Inference book (Parr, 2022) has been my (Xiq’s) main resource for this post. The book gives two paths into active inference: the “low road” which takes the Bayesian brain hypothesis as a given and expands Bayesian inference to include actions, and the “high road,” which makes as few assumptions as possible, starting only with the observation that things that don’t dissipate must have boundaries. It formally defines what things are, argues for how world models emerge, and explains how surprise minimization is basically a thermodynamic necessity.
I also spent a while surveying other ways people explained active inference and the FEP. I liked this lecture by Maxwell Ramstead. He motivates it as a way to make sense of nested multi-scale systems. He starts by saying that things must resist dissipation, then he explains predictive processing because the brain is a good central example, then Bayesian inference, and finally defines Markov blankets and variational free energy. He also wrote this Precis of the FEP.
I have also since found these two illustrated posts from 2018 which go into the math of the FEP a bit and its implementation as active inference quite a bit more.
This table of definitions for FEP/ActInf, Active Inference Ontology, is helpful for understanding all the terms and their relationships.
To learn more about Xiq’s work, which builds on the FEP and connects it to a wide range of ideas, see his Diagram, or our podcast conversation, Human Flourishing and Deep-Time (Audio / Video) where Xiq explains the diagram to Tasshin in detail.
You might also enjoy reading Xiq’s Free Energy Odyssey post, which he wrote in the process of research and writing this post.
Thank you to Kristijan Ivančić, Julian D’Costa, and River Kenna for reviewing this post.